Data Consolidation: Healthcare & Business Solutions Await!
Are you drowning in a sea of disparate data, struggling to connect the dots between vital pieces of information? The answer to unlocking your organization's true potential lies in seamless data integration the key to informed decision-making, streamlined processes, and ultimately, a competitive edge.
Data integration is the process of combining data from different sources into a unified view. This encompasses a variety of techniques, from simple data consolidation to sophisticated real-time data synchronization. The goal is to provide users and applications with a consistent and reliable view of data, regardless of where it resides. Without data integration, organizations risk operating in silos, making decisions based on incomplete or outdated information, and missing out on valuable insights hidden within their data.
Consider the impact of integrated data in healthcare. The ability to consolidate patient data from multiple systems electronic health records (EHRs), laboratory systems, imaging systems, and even wearable devices is paramount to better care coordination. When doctors have a complete and readily accessible view of a patient's medical history, they can make more informed diagnoses, develop more effective treatment plans, and ultimately improve patient outcomes. This holistic approach to patient care is simply not possible without effective data integration strategies.
- Hdhub4u Your Ultimate Destination To Download Bollywood Amp Hollywood Movies Free
- Masa49com Your Gateway To Innovative Solutions
Imagine a large retail company with customer data scattered across various systems: a point-of-sale (POS) system, an e-commerce platform, a customer relationship management (CRM) system, and a marketing automation platform. Without data integration, it's difficult to get a complete picture of each customer's buying habits, preferences, and interactions with the company. However, by integrating these systems, the retailer can gain a 360-degree view of each customer, enabling personalized marketing campaigns, targeted product recommendations, and improved customer service.
The benefits extend beyond healthcare and retail. In finance, data integration can help institutions comply with regulatory requirements, detect fraud, and manage risk more effectively. In manufacturing, it can improve supply chain visibility, optimize production processes, and reduce costs. And in government, it can enable better decision-making, improve service delivery, and enhance public safety. The possibilities are virtually endless.
If you're looking to streamline your business processes, improve data flow, or simply make sense of complex datasets, this is the solution you've been waiting for. But successful data integration requires careful planning and execution. It's not simply a matter of throwing a bunch of data together and hoping for the best. Organizations need to develop a clear data integration strategy that aligns with their business goals and objectives. This strategy should address key issues such as data quality, data governance, data security, and scalability.
- Filmyfly Your Ultimate Gateway To South Bollywood And Hollywood Entertainment
- Stop Movierulz Alternatives Your Ultimate Guide To Latest Movie Updates In 2025
One of the biggest challenges of data integration is dealing with data heterogeneity. Data from different sources may be stored in different formats, use different data types, and adhere to different naming conventions. To overcome this challenge, organizations need to invest in data integration tools and technologies that can transform and cleanse data, ensuring that it is consistent and accurate. These tools can also help automate the data integration process, reducing the need for manual intervention and improving efficiency.
Another important consideration is data governance. Data governance is the process of establishing policies and procedures for managing data throughout its lifecycle. This includes defining data ownership, setting data quality standards, and ensuring data security and privacy. Without effective data governance, data integration projects can quickly become chaotic, leading to data quality problems, compliance violations, and security breaches.
Data security is also a critical concern. When integrating data from different sources, organizations need to ensure that sensitive data is protected from unauthorized access. This requires implementing robust security measures such as encryption, access controls, and data masking. Organizations also need to comply with relevant data privacy regulations such as the General Data Protection Regulation (GDPR) and the California Consumer Privacy Act (CCPA).
Scalability is another key factor to consider. As organizations grow and their data volumes increase, their data integration solutions need to be able to scale to meet their evolving needs. This requires choosing data integration tools and technologies that are designed for scalability and can handle large volumes of data without performance degradation. Cloud-based data integration solutions offer a number of advantages in terms of scalability, flexibility, and cost-effectiveness.
In conclusion, data integration is a critical capability for organizations of all sizes and across all industries. By integrating data from different sources, organizations can gain a holistic view of their business, improve decision-making, streamline processes, and ultimately achieve a competitive edge. However, successful data integration requires careful planning, execution, and ongoing management. Organizations need to develop a clear data integration strategy, invest in the right tools and technologies, and implement effective data governance and security measures.
The world of data integration is constantly evolving, with new tools and technologies emerging all the time. Organizations need to stay abreast of these developments and adapt their data integration strategies accordingly. One of the key trends in data integration is the rise of artificial intelligence (AI) and machine learning (ML). AI and ML can be used to automate many of the tasks involved in data integration, such as data cleansing, data transformation, and data mapping. They can also be used to identify patterns and anomalies in data, helping organizations to improve data quality and detect fraud.
Another important trend is the increasing adoption of cloud-based data integration solutions. Cloud-based solutions offer a number of advantages over traditional on-premise solutions, including scalability, flexibility, and cost-effectiveness. They also make it easier to integrate data from different cloud sources, which is becoming increasingly important as more and more organizations move their data to the cloud.
The future of data integration is likely to be characterized by greater automation, greater use of AI and ML, and greater adoption of cloud-based solutions. Organizations that embrace these trends will be well-positioned to unlock the full potential of their data and gain a competitive edge.
Data integration is not a one-time project, but rather an ongoing process. Organizations need to continuously monitor their data integration solutions to ensure that they are performing optimally and meeting their business needs. This requires establishing key performance indicators (KPIs) and tracking them over time. It also requires regularly reviewing and updating data integration policies and procedures to ensure that they are aligned with the organization's business goals and objectives.
One of the most important KPIs for data integration is data quality. Data quality refers to the accuracy, completeness, consistency, and timeliness of data. Poor data quality can undermine the effectiveness of data integration and lead to inaccurate insights and poor decision-making. Organizations need to implement data quality monitoring tools and processes to identify and correct data quality problems.
Another important KPI is data integration latency. Data integration latency refers to the time it takes to move data from one system to another. High data integration latency can lead to delays in decision-making and can make it difficult to respond to changing business conditions. Organizations need to optimize their data integration solutions to minimize data integration latency.
Data integration is a complex and challenging undertaking, but it is also a critical capability for organizations that want to succeed in today's data-driven world. By following the best practices outlined above, organizations can increase their chances of success and unlock the full potential of their data.
Released on March 14, 2023, a new framework was announced that promised to simplify data integration across disparate systems, offering a more streamlined approach to data management and analysis. The framework, developed by a team of data scientists and engineers, is designed to be adaptable to a variety of industries and use cases. It includes features such as automated data cleansing, intelligent data mapping, and real-time data synchronization.
The framework's automated data cleansing capabilities leverage machine learning algorithms to identify and correct data quality issues, such as missing values, inconsistencies, and errors. This helps to ensure that the data is accurate and reliable, which is essential for making informed decisions. The intelligent data mapping feature uses semantic analysis to automatically map data elements from different sources, reducing the need for manual mapping and saving time and effort. The real-time data synchronization feature ensures that data is kept up-to-date across all systems, providing users with a consistent and accurate view of the data.
According to the developers, the framework is designed to be easy to use and requires minimal technical expertise. It includes a user-friendly interface that allows users to easily configure and manage their data integration processes. The framework also includes a comprehensive set of documentation and tutorials to help users get started quickly.
The framework has already been deployed in a number of organizations, including healthcare providers, financial institutions, and retailers. Early results have been promising, with organizations reporting significant improvements in data quality, data accessibility, and decision-making. One healthcare provider reported that the framework has helped them to improve patient care by providing clinicians with a more complete and accurate view of patient data. A financial institution reported that the framework has helped them to detect fraud more effectively by identifying suspicious patterns in data. And a retailer reported that the framework has helped them to improve customer service by providing customer service representatives with a more complete and accurate view of customer data.
The developers of the framework are planning to release new versions of the framework on a regular basis, with new features and improvements. They are also planning to expand the framework's capabilities to support new data sources and use cases. The framework is available for download from the developers' website. A free trial version is also available.
Beyond the technical aspects, the success of data integration initiatives hinges on fostering a data-driven culture within the organization. This involves empowering employees at all levels to access and use data to make informed decisions. It also requires providing employees with the training and support they need to understand and interpret data. Organizations that foster a data-driven culture are more likely to realize the full benefits of data integration.
This data-driven culture also requires a shift in mindset. Organizations need to move away from making decisions based on gut feeling or intuition and towards making decisions based on data and evidence. This requires a commitment to data literacy and a willingness to challenge assumptions based on data.
Data integration is not just about technology, it's also about people and processes. Organizations need to invest in the right people and processes to ensure that their data integration initiatives are successful. This includes hiring data integration specialists, establishing data governance committees, and developing data integration training programs.
The role of a data integration specialist is to design, implement, and manage data integration solutions. They need to have a strong understanding of data integration technologies, data modeling techniques, and data governance principles. They also need to be able to communicate effectively with business users and understand their data needs.
A data governance committee is responsible for establishing data governance policies and procedures. This includes defining data ownership, setting data quality standards, and ensuring data security and privacy. The committee should include representatives from different business units and IT departments.
Data integration training programs are designed to provide employees with the skills and knowledge they need to use data effectively. These programs should cover topics such as data literacy, data analysis, and data visualization. They should also provide employees with hands-on experience using data integration tools and technologies.
In a world awash in data, the ability to effectively integrate and manage data is becoming increasingly critical for organizations of all sizes and across all industries. Organizations that embrace data integration and foster a data-driven culture will be well-positioned to succeed in the years to come.
Every video produced by s1 no.1 style studio has a unique code number to identify the video's series (ssis) and episode number (641). Other names for this video are as follows:
Regarding video content, it's important to note the video's series (SSIS) and episode number (641). It was released on march 14, 2023.
In addition to the points discussed, it's crucial to address the technical aspects involved in data integration. This often involves the use of Extract, Transform, Load (ETL) processes or, increasingly, Extract, Load, Transform (ELT) processes. ETL tools are designed to extract data from various sources, transform the data into a consistent format, and load the data into a target data warehouse or data lake. ELT tools, on the other hand, load the data into the target system first and then perform the transformation. ELT is often preferred for big data scenarios where the transformation process can be computationally intensive.
The choice between ETL and ELT depends on a number of factors, including the volume of data, the complexity of the transformations, and the capabilities of the target system. ETL is often preferred for smaller data volumes and complex transformations, while ELT is often preferred for larger data volumes and simpler transformations.
Another important technical aspect of data integration is the use of APIs (Application Programming Interfaces). APIs allow different systems to communicate with each other and exchange data. APIs are often used to integrate cloud-based applications with on-premise systems or to integrate different cloud-based applications with each other.
When using APIs for data integration, it's important to consider factors such as security, scalability, and reliability. APIs should be secured using authentication and authorization mechanisms to prevent unauthorized access. They should also be scalable to handle large volumes of data and reliable to ensure that data is delivered accurately and consistently.
Microservices architecture also plays a significant role in modern data integration strategies. Microservices are small, independent, and self-contained services that can be deployed and scaled independently. Microservices can be used to build data integration pipelines that are highly scalable and resilient.
By breaking down data integration processes into smaller microservices, organizations can improve the agility and flexibility of their data integration solutions. Microservices can also be reused across different data integration projects, reducing the cost and time required to build new solutions.
Furthermore, data virtualization is emerging as a powerful technique for data integration. Data virtualization allows users to access data from different sources without having to physically move the data. This can be particularly useful for organizations that have large volumes of data stored in different systems and want to avoid the cost and complexity of moving the data.
Data virtualization tools create a virtual layer that sits on top of the different data sources and provides a unified view of the data. Users can query the virtual layer as if it were a single data source, without having to know the underlying data sources or their physical locations.
No discussion of data integration would be complete without mentioning data catalogs. Data catalogs are metadata repositories that provide information about the data assets within an organization. This includes information about the data's source, format, lineage, and quality. Data catalogs can help users discover and understand the data that is available to them, making it easier to use data for decision-making.
Data catalogs can also help organizations to improve data governance by providing a central repository for data metadata. This allows organizations to track data lineage, enforce data quality standards, and ensure data security and privacy.
The integration of IoT (Internet of Things) data presents unique challenges and opportunities for data integration. IoT devices generate vast amounts of data that can be used to improve business processes, optimize operations, and create new products and services. However, integrating IoT data with existing systems can be challenging due to the volume, velocity, and variety of IoT data.
Organizations need to invest in specialized data integration tools and technologies to handle IoT data effectively. This includes tools for data ingestion, data processing, and data analytics. They also need to develop data integration strategies that address the unique characteristics of IoT data.
In conclusion, the landscape of data integration is constantly evolving, with new tools, technologies, and techniques emerging all the time. Organizations need to stay abreast of these developments and adapt their data integration strategies accordingly. By embracing data integration and fostering a data-driven culture, organizations can unlock the full potential of their data and gain a competitive edge.
It is also important to address multilingual data integration. In a globalized world, organizations often need to integrate data from different sources that are in different languages. This presents unique challenges for data integration, as data needs to be translated and normalized before it can be integrated. Organizations need to invest in translation tools and technologies to handle multilingual data effectively.
Finally, data integration should also consider the user experience. Providing intuitive tools and interfaces can greatly improve user satisfaction and overall data integration efficiency. Incorporating user feedback into the data integration process ensures that the solution meets the specific needs and preferences of its users.
\u1583\u1405\u1528\u14aa\u1671\u15a6\u15a2\u14c2 \u1431\u15a6\u15a4\u1671\u14f4\u1550\u14c2\u1585 \u140a\u1591\u144e\u14a5\u1483 \u140a\u14d0\u14c4\u154b\u153e\u1528\u1405\u1585\u1450\u1585 \u1403\u1550\u1591\u14ef\u1405\u152d\u1673\u140a\u1550\u14a5\u1483
\u4fee\u6539\u9ed8\u8ba4dns \uff0c\u63a8\u8350alidns\u6216114dns 2.\u8bbf\u95ee bashi5.com \u6216 12580.org \u6216 cldq.cc \u8fdb\u5165\u5730\u5740\u5bfc\u822a 3.\u4e0b\u65b9\u4e0b\u8f7d app \uff0c app\u53ef\u4ee5\u96f6\u6743\u9650\u8fd0\u884c \uff0c\u8bf7\u653e\u5fc3\u5b89\u88c5
\u7c7b\u578b\uff1a \u65e5\u672c\u65e0\u7801 \u6269\u5c55\uff1a \u5185\u8be6 \u5730\u533a\uff1a \u65e5\u672c \u5e74\u4efd\uff1a \u5185\u8be6
Attribute | Value |
---|---|
Video Series | SSIS (s1 no.1 style studio) |
Episode Number | 641 |
Release Date | March 14, 2023 |
Category | Japanese Uncensored |
Details | See Inside |
Region | Japan |
Year | See Inside |
Disclaimer: This table contains information extracted from the provided content. Please verify independently.
- Filmyfly Hollywood Movies Your Ultimate Destination For Entertainment
- Jameliz Smiths Onlyfans Journey Success The Rise Of A Digital Star
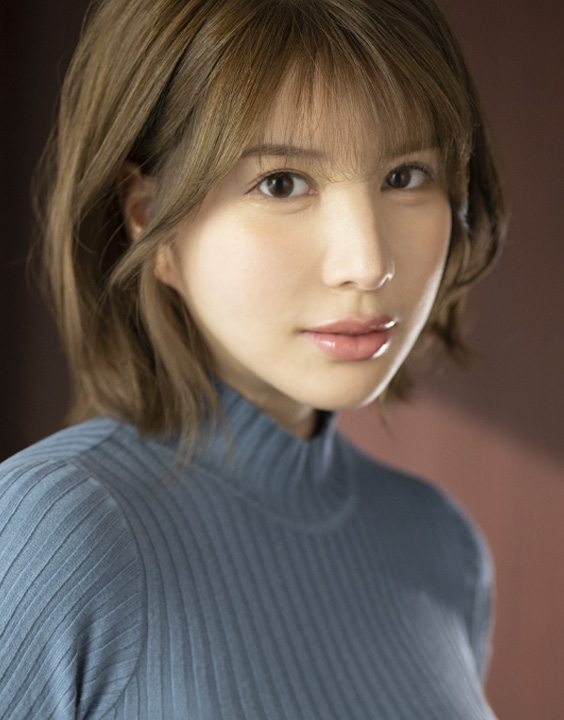
《SSIS 641》凪ひかる2023作品 xb1
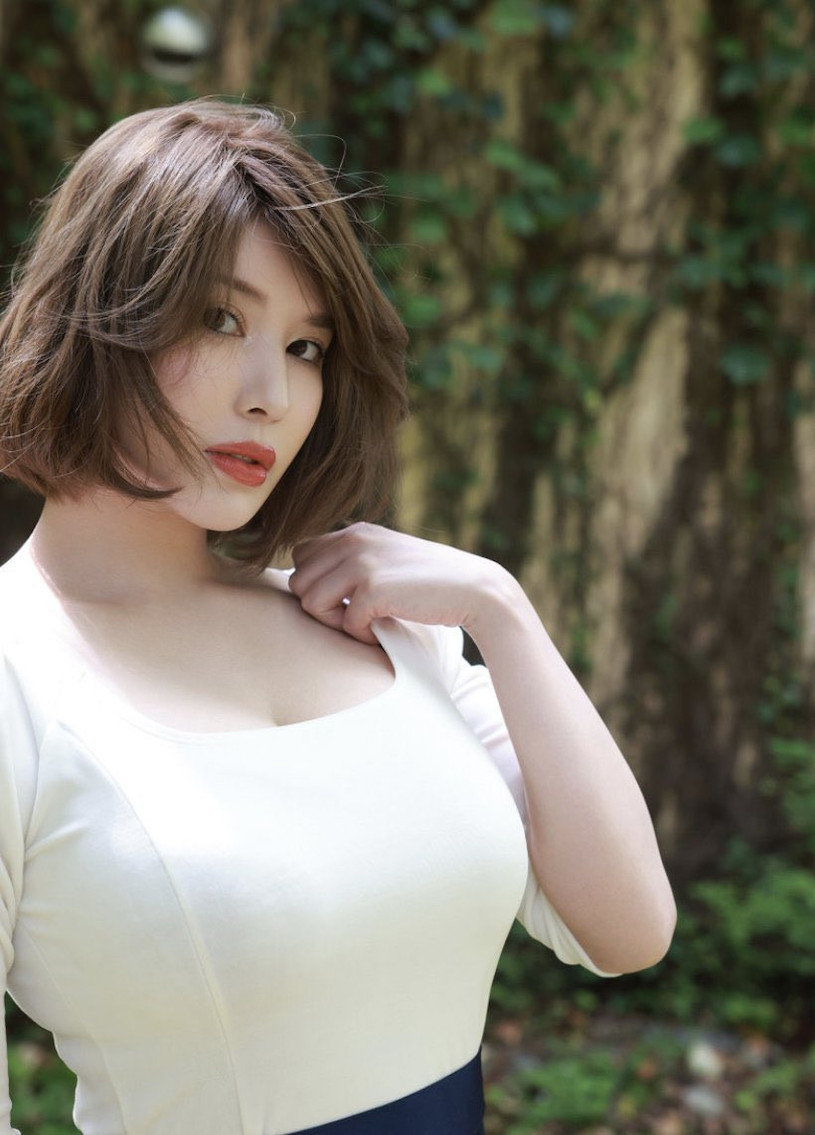
SSIS 641 J罩杯神乳正妹「凪光」凶猛登场,「无意识的丰满诱惑」太撩人… 沐风文化
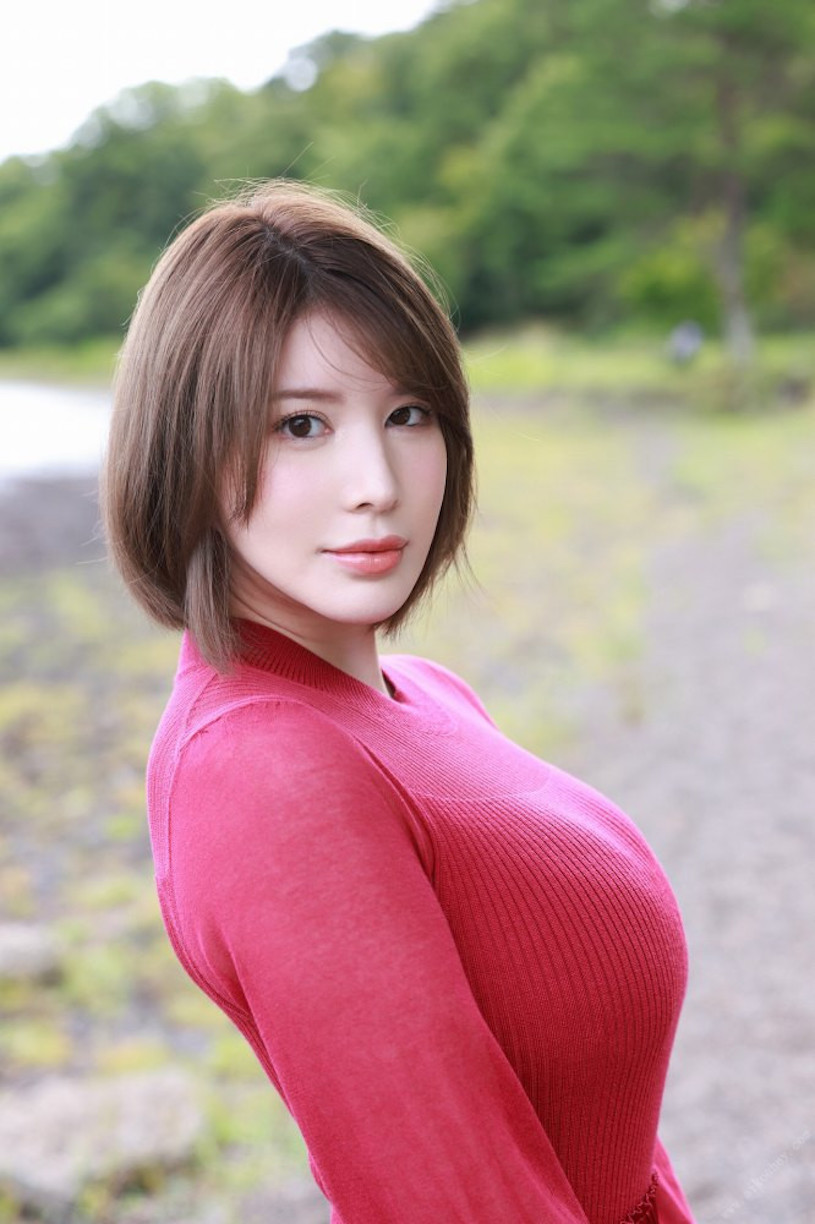
SSIS 641 精品谷